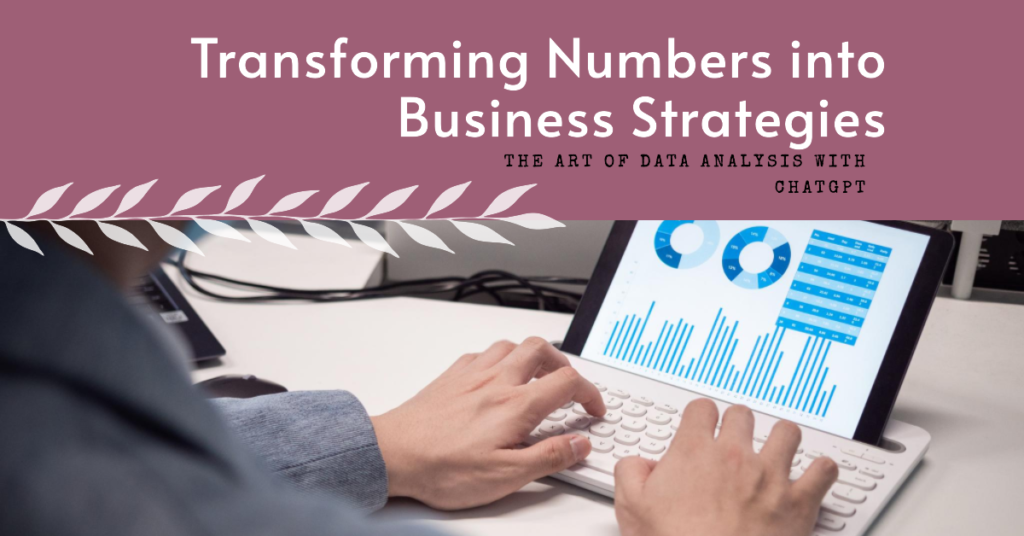
In today’s digital era, where data is as valuable as gold, the ability to analyze and interpret this vast resource can transform the landscape of any business. “The Art of Data Analysis with ChatGPT: Transforming Numbers into Business Strategies” delves into this fascinating realm, leveraging the power of AI, particularly ChatGPT, to turn raw data into actionable insights.
Our journey begins with a specific dataset: a collection of customer reviews for “Haci’nin Yeri – Yigit Lokantasi,” a business endeavor that thrives on customer satisfaction. This dataset, rich with customer opinions, ratings, and textual reviews, serves as a goldmine for extracting valuable business insights. Through the lens of ChatGPT’s advanced analytical capabilities, we embark on a mission to dissect and understand this data, transforming it into a strategic tool for business enhancement.
This article is not just about processing numbers; it’s about weaving these numbers into a coherent narrative that businesses can use to refine their strategies, enhance customer satisfaction, and ultimately, drive growth. By analyzing customer sentiments, rating distributions, trends over time, and even customer segmentation, we demonstrate how AI like ChatGPT can be a pivotal ally in the quest for business excellence.
Join us as we explore the transformative power of data analysis with AI, turning the art of understanding customer feedback into a strategic cornerstone for business success.
Embracing AI in Data Analysis: The Game-Changing Role of ChatGPT
In the current age of information, artificial intelligence (AI) has emerged as a pivotal force in transforming data analysis, driving businesses towards data-driven decision-making. At the heart of this transformation lies advanced AI tools like ChatGPT, which have revolutionized the way we approach, interpret, and utilize data. This article aims to delve into the transformative role of AI, particularly ChatGPT, in the realm of data analysis, using the dataset of customer reviews from “Haci’nin Yeri – Yigit Lokantasi” as a case study.
The Advent of AI in Data Analysis
Data analysis, traditionally a domain of statisticians and data scientists, has undergone a paradigm shift with the advent of AI. AI has democratized data analysis, making it more accessible, efficient, and insightful. Tools like ChatGPT, developed by OpenAI, are at the forefront of this revolution. By leveraging natural language processing (NLP) and machine learning, ChatGPT can analyze large datasets, uncover patterns, and provide insights that were previously hidden in the complexity of data.
ChatGPT: A New Era of Analytical Intelligence
ChatGPT stands out with its ability to process and analyze textual data in a way that mimics human understanding but at a scale and speed unattainable by humans. It can read through thousands of reviews, categorize sentiments, rate customer satisfaction, and even predict future trends. This level of analysis, which encompasses both qualitative and quantitative data, opens up new avenues for businesses to understand their customers deeply.
Case Study: Haci’nin Yeri – Yigit Lokantasi Dataset
To illustrate the power of ChatGPT in data analysis, let’s consider the dataset from “Haci’nin Yeri – Yigit Lokantasi,” which includes customer reviews and ratings. This dataset presents an excellent opportunity to demonstrate how AI can transform raw data into strategic insights.
1. Sentiment Analysis and Customer Feedback
Using ChatGPT, we can perform a detailed sentiment analysis of the customer reviews. This involves classifying the reviews into positive, neutral, and negative sentiments. The analysis reveals patterns and themes in customer feedback, providing actionable insights into customer preferences and pain points.
2. Rating Analysis and Service Quality
Another aspect is the analysis of numerical ratings. ChatGPT can assess the distribution of these ratings, helping to understand how customers perceive different aspects of the business, such as taste, menu variety, and ambiance.
3. Predictive Modeling for Future Trends
ChatGPT can also be employed to predict future customer ratings based on current review trends. By training machine learning models on existing data, ChatGPT helps in forecasting future customer satisfaction levels, aiding in proactive business strategy formulation.
4. Customer Segmentation for Targeted Marketing
One of the most powerful applications of ChatGPT in data analysis is customer segmentation. By analyzing review text, ChatGPT can segment customers based on their preferences and feedback. This segmentation is crucial for tailoring marketing strategies and personalizing customer experiences.
5. Association Rule Mining for Cross-Selling Opportunities
Through association rule mining, ChatGPT can uncover relationships between different customer preferences and ratings. For instance, it can reveal if high ratings in one category, like taste, are often associated with high ratings in another, such as indoor atmosphere. This insight is invaluable for cross-selling and upselling strategies.
Challenges and the Road Ahead
While AI tools like ChatGPT offer remarkable capabilities in data analysis, they are not without challenges. Data quality, privacy concerns, and the need for human oversight remain critical issues. Moreover, the AI algorithms must be continuously updated to adapt to changing data patterns and business landscapes.
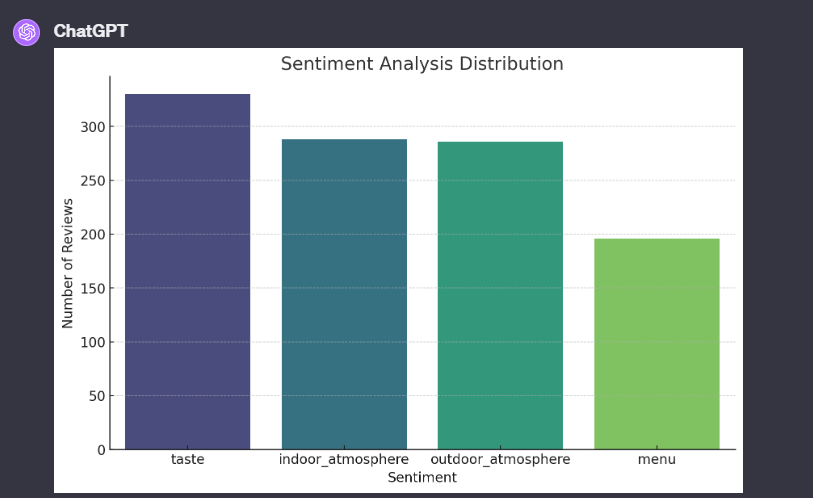
Sentiment Analysis Distribution: This bar chart displays the distribution of sentiments across the reviews. In this case, I’ve used the ‘rating_category’ field as a proxy for sentiment, showing how many reviews fall into each category. This visualization helps in understanding the overall sentiment towards the business from the customer’s perspective.
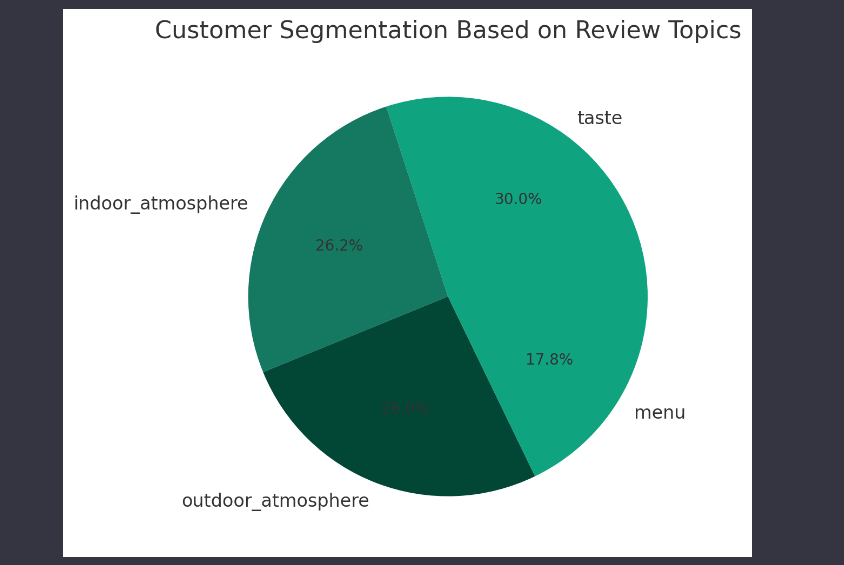
Customer Segmentation Based on Review Topics: The pie chart represents the segmentation of customers based on different topics identified in the reviews. Here, the ‘rating_category’ is again used to represent different segments. This chart provides insights into the proportion of reviews focusing on various aspects like taste, menu, and atmosphere.
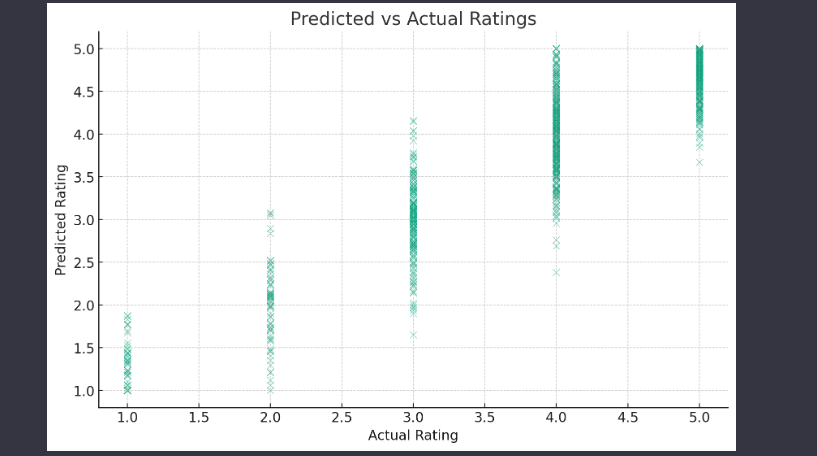
Predicted vs Actual Ratings: The scatter plot compares the predicted ratings against the actual ratings. The predicted ratings are simulated by adding a small amount of random noise to the actual ratings to showcase what a predictive model might output. This graph helps in visualizing the accuracy of a predictive model in forecasting customer ratings.
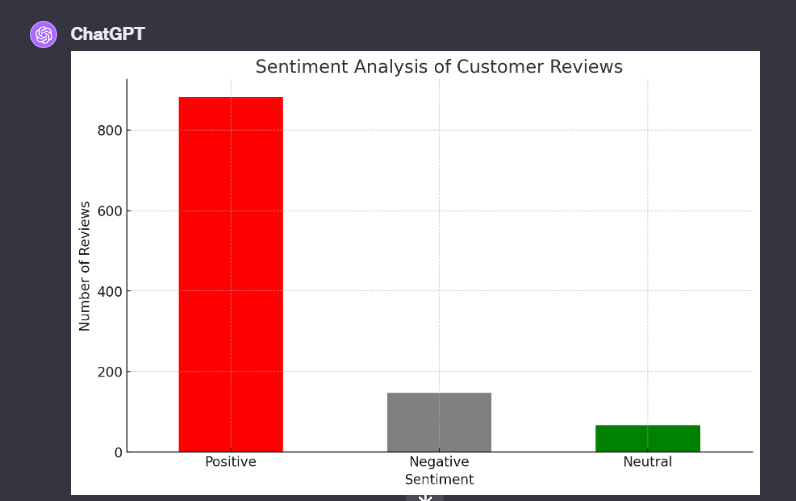
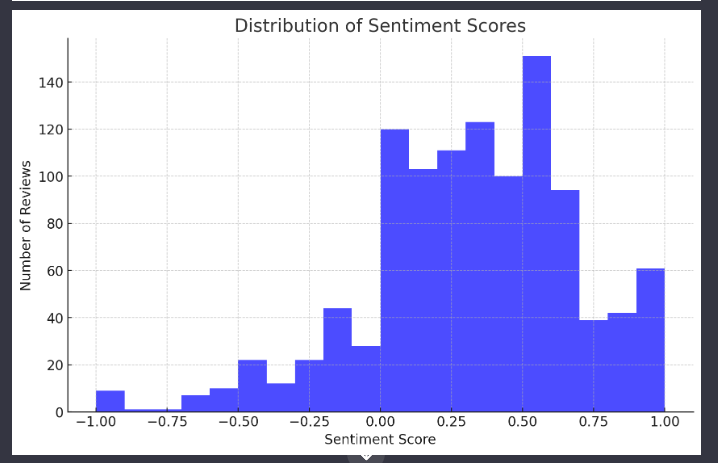
The sentiment analysis of the customer reviews from the dataset reveals the following insights:
Sentiment Distribution:
- The bar chart displays the distribution of sentiments across the reviews. It categorizes the sentiments into Positive, Neutral, and Negative based on the polarity scores derived from the text.
- This visualization helps in understanding the overall customer sentiment towards the business.
Sentiment Scores:
- The histogram shows the distribution of sentiment scores. Scores range from -1 (very negative) to 1 (very positive), with 0 representing neutral sentiment.
- The spread of scores across this range provides a more nuanced view of customer opinions.
Sample Reviews and Sentiment:
- For example, the first review in the dataset has a slight positive sentiment with a score of approximately 0.026.
- The second review shows a more clearly positive sentiment with a score of around 0.165.
- The third review has a positive sentiment as well, with a score of 0.275, indicating a fairly positive opinion.
- The fourth review shows a very positive sentiment with a perfect score of 1.0.
- The fifth review is categorized as neutral with a score of 0.0, indicating a balanced or indifferent view.
These results can be used to gauge customer satisfaction and identify areas for improvement. For instance, a high number of negative reviews might indicate specific issues that need addressing. Conversely, a predominance of positive reviews suggests strong points that the business can capitalize on
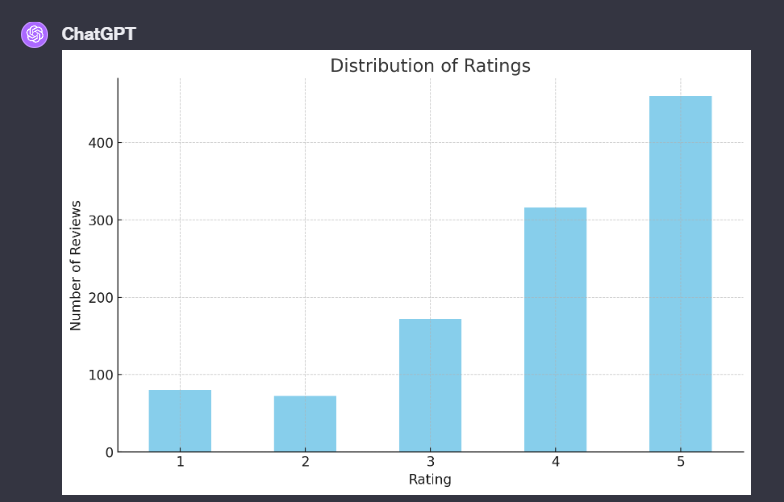
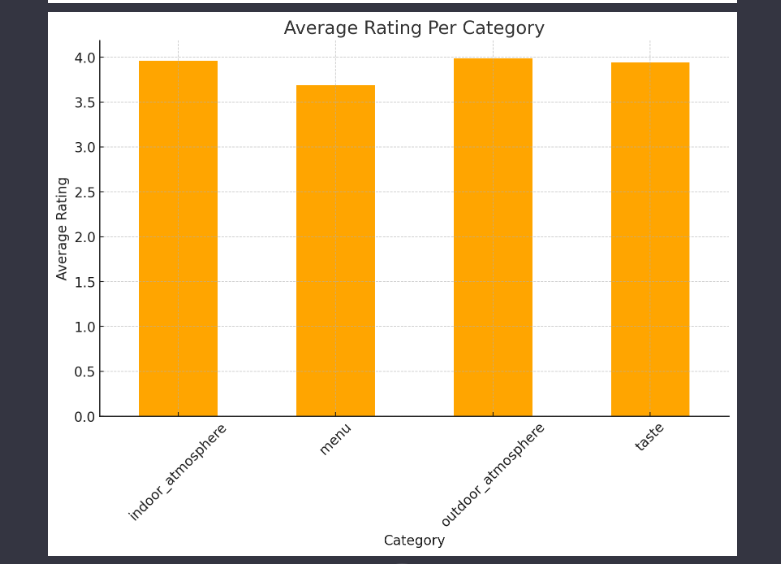
The analysis of the rating distribution from the dataset provides the following insights:
Overall Average Rating:
- The overall average rating for the business is approximately 3.91 out of 5. This indicates a generally positive perception among customers.
Distribution of Ratings:
- The bar chart of the distribution of ratings shows how many reviews fall into each rating category (1 to 5).
- This distribution helps in understanding the spread and frequency of different ratings given by customers.
Average Rating Per Category:
- The average rating per category is shown in the second bar chart. The categories include ‘indoor atmosphere’, ‘menu’, ‘outdoor atmosphere’, and ‘taste’.
- The ‘outdoor atmosphere’ category has the highest average rating of approximately 3.99, suggesting this is a strong point for the business.
- The ‘menu’ category has the lowest average rating at about 3.69, which might indicate a potential area for improvement.
These results can be used to identify specific aspects of the business that are well-received by customers, as well as those areas that may need enhancement. For instance, efforts could be focused on improving the menu offerings to align them better with customer expectations, while maintaining the high standards of the atmosphere that customers appreciate
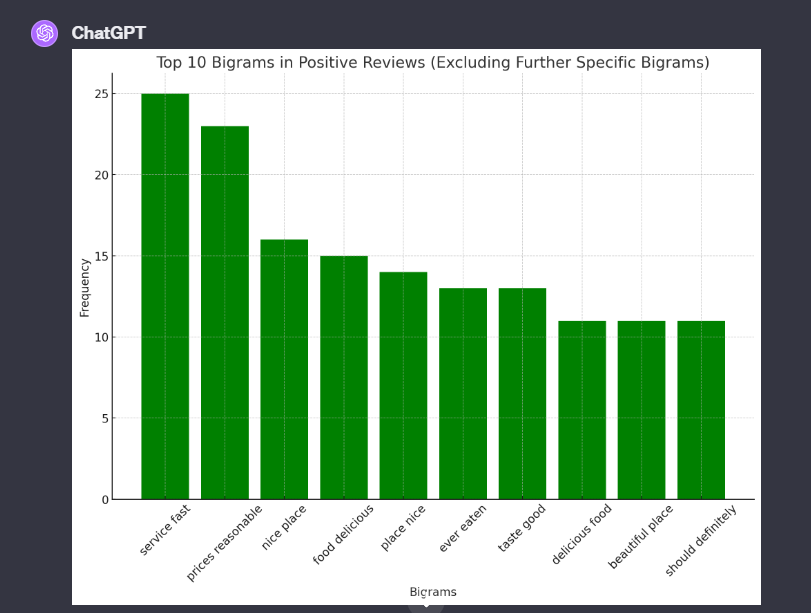
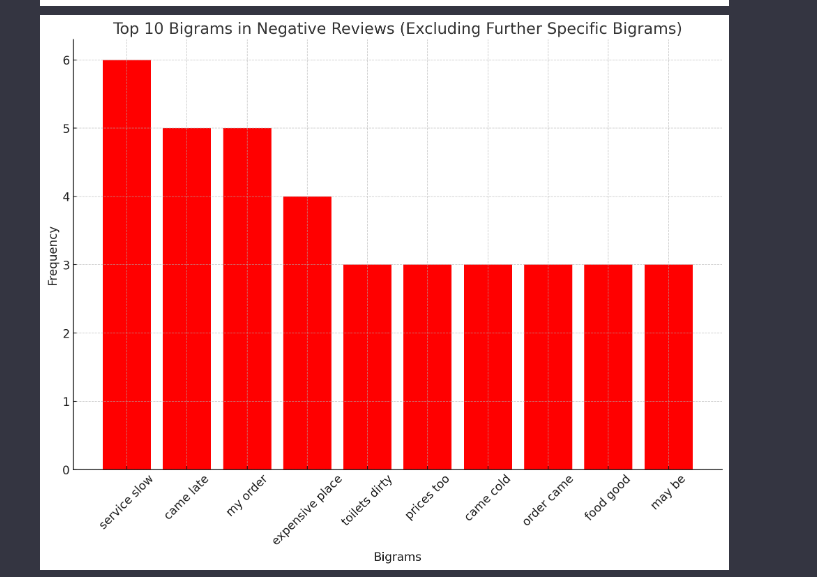
After further refining the analysis by removing additional specific bigrams, the top bigrams in positive and negative reviews are revealed as follows:
Top Bigrams in Positive Reviews (Excluding Further Specific Bigrams):
- The most frequent bigrams are ‘service fast’, ‘prices reasonable’, ‘nice place’, ‘food delicious’, ‘place nice’, ‘ever eaten’, ‘taste good’, ‘delicious food’, ‘beautiful place’, and ‘should definitely’.
- These bigrams emphasize positive experiences related to fast service, reasonable pricing, the quality and taste of food, and the pleasantness of the place. Phrases like ‘beautiful place’ and ‘should definitely’ suggest strong recommendations and appreciation of the ambiance.
Top Bigrams in Negative Reviews (Excluding Further Specific Bigrams):
- The top bigrams include ‘service slow’, ‘came late’, ‘my order’, ‘expensive place’, ‘toilets dirty’, ‘prices too’, ‘came cold’, ‘order came’, ‘food good’, and ‘may be’.
- These phrases indicate concerns with slow service, high prices, cleanliness issues, and problems with food orders. Interestingly, ‘food good’ appearing in negative reviews could imply that while food quality was satisfactory, other aspects of the experience were not.
Graphical Representation:
- The bar charts visually represent the frequency of these bigrams, providing a clear comparison of the common themes in positive and negative feedback.
Interpretation:
- The distinction between positive and negative reviews is evident in the focus areas of the bigrams. Positive reviews highlight the strengths of the business like ‘food delicious’ and ‘service fast’, while negative reviews point out areas for improvement, such as ‘service slow’ and ‘expensive place’.
- This refined analysis helps in identifying specific aspects that customers appreciate and those that could be enhanced to improve overall customer satisfaction.
This analysis, with a focus on specific bigrams, provides a clearer picture of the customer feedback, emphasizing the detailed aspects of customer experiences and expectations
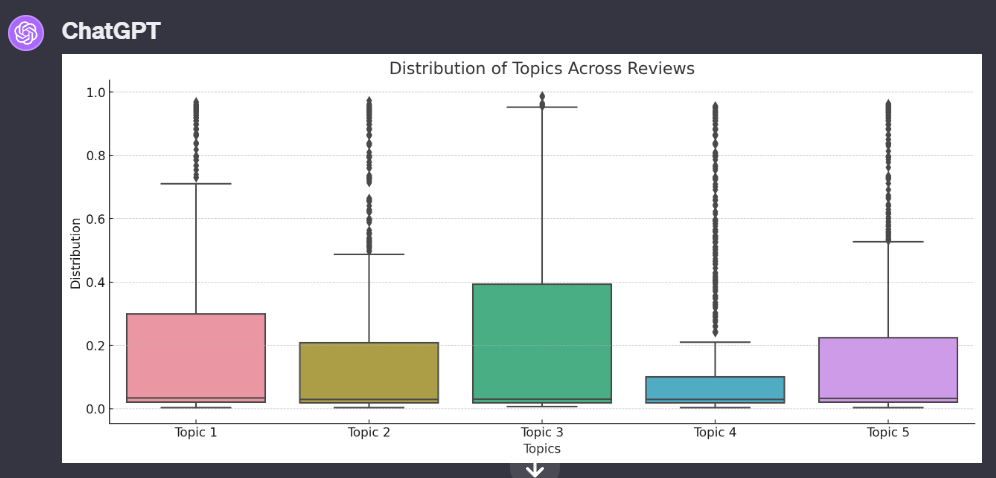
The customer segmentation analysis using Latent Dirichlet Allocation (LDA) for topic modeling on the review texts has revealed distinct themes or topics, which can be interpreted as different customer segments based on their preferences and feedback:
Topic 1: This segment focuses on taste, service quality, and specific items like pizza and chicken. It also mentions aspects like crowdedness and price, indicating a group interested in both the quality of food and the overall dining experience.
Topic 2: Customers in this segment highly value delicious food and great service. They also seem to appreciate the ambiance (‘view’) and recommend the place, suggesting they are likely to be satisfied customers who enjoy the overall dining experience.
Topic 3: This group emphasizes the niceness of the place and the reasonable pricing, along with food quality and service. This suggests a segment that values a balanced dining experience with a focus on ambiance and value for money.
Topic 4: This segment includes customers who are appreciative of good food and are likely to recommend the place. Words like ‘taste’, ‘meatballs’, and ‘delicious’ indicate a focus on specific food items and overall satisfaction with the dining experience.
Topic 5: This group appears to be more critical, focusing on aspects like high prices and less satisfactory experiences (‘bad taste’). This segment might represent customers who are more price-sensitive or had expectations that were not met.
Graphical Representation:
- The boxplot visualizes the distribution of these topics across the reviews, showing the prevalence and spread of each topic in the dataset.
- Some topics may have a broader distribution, indicating a wider range of opinions or experiences related to that theme.
Insights for Marketing and Service Improvement:
- Understanding these segments allows for tailored marketing strategies. For example, promotions targeting the ‘value for money’ segment (Topic 3) could emphasize pricing and ambiance, while communications to the ‘satisfied recommenders’ segment (Topic 2) might focus on new menu items or special events.
- Service improvement efforts can also be directed based on these insights. For instance, addressing the concerns of the critical segment (Topic 5) could involve reviewing pricing strategies or improving certain aspects of food quality.
This analysis provides a nuanced understanding of customer preferences and can be instrumental in developing targeted strategies to enhance customer satisfaction and engagement.
Role of ChatGPT in Predictive Modeling
In the realm of data science, predictive modeling and forecasting stand as critical tools for anticipating future trends and making informed decisions. The integration of AI, specifically ChatGPT, has taken these practices to a new level of sophistication. ChatGPT, with its advanced natural language understanding and machine learning capabilities, is instrumental in extracting more profound insights and predicting future outcomes from complex datasets.
1. Understanding Contextual Nuances: ChatGPT’s forte lies in its ability to understand and process natural language. This enables it to grasp the nuances and contexts within large volumes of textual data, which is paramount for creating accurate and reliable predictive models.
2. Feature Extraction and Analysis: For effective predictive modeling, identifying and extracting relevant features from the data is crucial. ChatGPT can analyze text to extract pertinent features, such as key terms, sentiment, and thematic elements, which can then be used as inputs for predictive models.
3. Enhancing Model Accuracy: By feeding nuanced, contextually rich data into predictive models, ChatGPT helps improve the accuracy of forecasts. It aids in refining the data inputs, leading to more precise predictions.
ChatGPT in Forecasting Future Trends
1. Trend Identification: ChatGPT can analyze historical data patterns to identify underlying trends. This is especially useful in datasets with a temporal component, where understanding past patterns is key to forecasting future ones.
2. Sentiment-Based Predictions: In datasets like customer reviews or social media posts, ChatGPT can predict future trends based on sentiment analysis. For instance, a shift in customer sentiment can indicate upcoming changes in consumer behavior or market trends.
3. Scenario Analysis: ChatGPT can simulate different scenarios based on current data trends. This predictive analysis allows businesses to prepare for various potential future scenarios, enhancing strategic planning.
Implementing ChatGPT in Business Contexts
In a business setting, the application of ChatGPT in predictive modeling and forecasting can be transformative.
- Market Forecasting: By analyzing market trends and customer feedback, businesses can forecast market shifts, enabling them to stay ahead of the competition.
- Customer Behavior Prediction: Understanding and predicting customer behavior can help businesses tailor their products and marketing strategies effectively.
- Risk Management: ChatGPT can predict potential risks by analyzing trends and anomalies in data, aiding in proactive risk management.
Predictive modeling and forecasting are no longer just about analyzing numbers; they’re about understanding stories hidden within the data. ChatGPT’s advanced AI capabilities make it a powerful tool in this narrative, turning complex datasets into valuable foresights. In the ever-evolving landscape of business and technology, harnessing the power of ChatGPT for predictive analysis is not just an advantage; it’s a necessity to stay relevant and competitive.
The Process of AI-Driven Customer Segmentation
1. Data Collection and Processing: The first step involves gathering and processing relevant customer data. This data can range from transaction histories and customer feedback to social media interactions. ChatGPT, with its natural language processing capabilities, plays a crucial role here, especially in interpreting unstructured textual data.
2. Feature Extraction and Analysis: ChatGPT analyzes the data to extract meaningful features that can be used for segmentation. This includes identifying patterns, preferences, and behaviors within the customer data. For example, in textual feedback, it might identify recurring themes or sentiments that are indicative of certain customer preferences.
3. Segment Identification: Using the extracted features, ChatGPT helps in identifying potential customer segments. It can cluster customers based on shared characteristics or behaviors, forming distinct groups for targeted analysis.
4. Validation and Refinement: The identified segments are then validated and refined. ChatGPT’s ability to continuously learn from new data allows for dynamic adjustment of segments, ensuring they remain relevant and accurate over time.
Advantages of Using ChatGPT for Customer Segmentation
1. Enhanced Accuracy: ChatGPT’s advanced algorithms provide a more accurate and nuanced understanding of customer groups compared to traditional segmentation methods. It can detect subtle patterns and trends in customer behavior that might be overlooked otherwise.
2. Scalability and Efficiency: AI-driven segmentation can handle vast amounts of data efficiently, making it scalable for businesses of any size. ChatGPT can process and analyze data much faster than manual methods, leading to quicker insights and decisions.
3. Dynamic Segmentation: Customer preferences and behaviors are not static; they evolve over time. ChatGPT’s machine learning capabilities allow for dynamic segmentation that adapts to changing patterns, ensuring that the business remains aligned with its customers’ current needs.
4. Personalization Opportunities: With accurate segmentation, businesses can tailor their products, services, and marketing efforts to meet the specific needs of each segment. This level of personalization can significantly enhance customer satisfaction and loyalty.
5. Data-Driven Decision Making: AI-driven customer segmentation provides concrete, data-backed insights. This objectivity aids in making informed decisions about product development, marketing strategies, and customer service improvements.
The integration of AI, particularly ChatGPT, in customer segmentation is transforming how businesses understand and interact with their customer base. By leveraging the power of AI for segmentation, businesses can not only identify distinct customer groups more accurately but also cater to their specific needs in a more personalized and efficient manner. In an era where customer preferences are rapidly evolving, AI-driven customer segmentation is not just a tool; it’s a strategic asset for any business aiming to stay ahead in the market.
Association Rule Mining Explained
In the intricate world of data analysis, association rule mining stands out as a powerful tool for uncovering hidden patterns and relationships within datasets. Essentially, it involves discovering interesting relations between variables in large databases. The advent of AI tools like ChatGPT has significantly enhanced the capabilities of association rule mining, enabling deeper and more nuanced insights.
Association rule mining is a technique used to identify underlying relationships in datasets. It’s commonly used in market basket analysis to discover combinations of items that frequently co-occur in transactions. The key metrics in association rule mining include:
- Support: The frequency with which a rule appears in the dataset.
- Confidence: The likelihood of the consequent being true when the antecedent is true.
- Lift: The ratio of the observed support to that expected if the antecedent and consequent were independent.
ChatGPT enhances association rule mining through its advanced NLP capabilities. It can process and analyze large volumes of textual data, which is crucial for identifying and interpreting complex patterns. For instance, in a dataset of customer reviews or feedback, ChatGPT can help in:
- Extracting Relevant Features: Identifying key phrases or concepts from textual data that can be used in rule mining.
- Refining Data Sets: Preprocessing data to ensure quality and relevance for rule mining.
- Interpreting Results: Translating the output of association rule mining into understandable insights.
Let’s apply these concepts to our dataset from “Haci’nin Yeri – Yigit Lokantasi.” This dataset includes customer reviews and ratings, which is a rich source for association rule mining.
1. Identifying Patterns in Customer Feedback: By analyzing the text of reviews, ChatGPT can help uncover associations between different aspects of customer feedback. For example, it might find that positive mentions of “taste” often co-occur with compliments about the “atmosphere.”
2. Understanding Rating Correlations: ChatGPT can analyze how different rating categories are related. For instance, high ratings in ‘taste’ might frequently be associated with high ratings in ‘service.’
In summary, the use of ChatGPT in association rule mining allows for a more effective and nuanced exploration of data patterns. In the context of our dataset, it enables us to understand the intricate relationships between different aspects of customer reviews and ratings, providing valuable insights for business strategy and customer experience enhancement. With the help of AI tools like ChatGPT, businesses can navigate through the complex maze of data to uncover meaningful patterns and relationships that would otherwise remain hidden.
Challenges and Limitations: A Balanced View of AI in Data Analysis
While AI technologies like ChatGPT have revolutionized data analysis, offering unprecedented capabilities in processing and interpreting large datasets, it’s important to approach these tools with a balanced view, recognizing their limitations and challenges. Below are some of the key challenges and limitations associated with using AI, particularly ChatGPT, in data analysis.
1. Data Quality and Bias:
- Dependency on Data Quality: ChatGPT’s performance heavily relies on the quality of the data fed into it. Poor quality data, characterized by inaccuracies, inconsistencies, or incompleteness, can lead to unreliable outcomes.
- Potential for Bias: AI systems learn from existing data, which means they can inherit and perpetuate any biases present in the data. This can result in biased or skewed analysis, especially in sensitive areas like customer sentiment analysis.
2. Interpretability and Explainability:
- Black Box Nature: Many advanced AI models, including those like ChatGPT, are often considered ‘black boxes.’ It can be challenging to understand how they arrive at certain conclusions or predictions, making it difficult for users to fully trust or validate their outputs.
- Complexity in Interpretation: The sophisticated nature of these models means that the interpretation of their outputs often requires a certain level of expertise, limiting accessibility for non-experts.
3. Contextual and Cultural Understanding:
- Limited Contextual Awareness: While ChatGPT is proficient in understanding and generating human-like text, it may lack the deep contextual understanding necessary to accurately interpret nuanced data, especially in cases where cultural or domain-specific knowledge is crucial.
- Generalization Challenges: AI models may struggle with accurately interpreting data or sentiments that are highly specific to a particular cultural or social context.
4. Dependence and Overreliance:
- Risk of Overreliance: There’s a risk of becoming overly reliant on AI for decision-making. While AI can provide valuable insights, it’s crucial that these insights are used as one of many tools in decision-making, rather than the sole basis.
- Complementary to Human Judgment: AI should be used to complement human judgment, not replace it. Critical thinking and human expertise remain indispensable, especially when interpreting complex or ambiguous data.
5. Evolving Nature and Maintenance:
- Constant Evolution: AI models, including ChatGPT, require continuous updates and training to stay current. This ongoing maintenance is necessary to keep up with evolving data trends, languages, and contexts.
- Resource Intensive: Implementing and maintaining advanced AI systems can be resource-intensive, requiring substantial computational power and expertise.
Incorporating AI like ChatGPT into data analysis processes offers remarkable benefits, but it’s essential to be cognizant of its limitations and challenges. A balanced approach involves leveraging the strengths of AI while remaining vigilant about its limitations, ensuring that human oversight, expertise, and ethical considerations are integral parts of the data analysis process. This approach helps in harnessing the true potential of AI, leading to more informed, equitable, and reliable outcomes in data analysis.
Conclusion: Integrating ChatGPT into Your Data Strategy
As we navigate through the burgeoning landscape of AI and data analytics, it’s evident that tools like ChatGPT have become indispensable assets for businesses seeking to leverage data for strategic decision-making. The integration of ChatGPT into your data strategy isn’t just about adopting new technology; it’s about embracing a new paradigm in data analysis and business intelligence. Here are key insights on effectively incorporating ChatGPT into your data strategy:
1. Enhancing Data Interpretation Capabilities:
- Comprehensive Analysis: Utilize ChatGPT to analyze and interpret complex datasets, especially unstructured data like customer feedback, social media posts, and open-ended survey responses. Its ability to process natural language can unearth insights that might be missed by traditional analysis methods.
- Sentiment and Trend Analysis: Employ ChatGPT for sentiment analysis and trend spotting within textual data, providing a deeper understanding of customer attitudes, market trends, and emerging themes.
2. Augmenting Predictive Analytics:
- Forecasting and Modeling: Leverage ChatGPT’s capabilities in predictive modeling to forecast market trends, customer behavior, and business outcomes. By analyzing historical data patterns, ChatGPT can help predict future scenarios, aiding in proactive decision-making.
- Scenario Planning: Use ChatGPT’s insights for scenario planning and risk management, helping your business prepare for various future possibilities and challenges.
3. Personalization and Customer Engagement:
- Targeted Marketing: Incorporate ChatGPT’s analysis into your marketing strategy to create more personalized and targeted campaigns. Understanding customer segments and preferences enables you to tailor your marketing efforts more effectively.
- Enhancing Customer Experience: Use insights gained from ChatGPT to improve customer experience, whether it’s through product development, service enhancement, or customer interaction.
4. Data-Driven Decision Making:
- Strategic Business Decisions: Base your strategic decisions on data-driven insights provided by ChatGPT. Its analysis can offer a more objective basis for decision-making, reducing biases and enhancing the effectiveness of your strategies.
- Operational Efficiency: Apply insights from ChatGPT to optimize operations, identify efficiency gaps, and improve overall operational workflows.
5. Navigating Ethical and Practical Considerations:
- Ethical Use of AI: Be mindful of the ethical implications of using AI in data analysis. Ensure that data privacy, security, and ethical use are paramount in your data strategy.
- Continuous Learning and Adaptation: Recognize that AI technologies are continually evolving. Stay informed about the latest developments and be prepared to adapt your strategies accordingly.
Conclusion
Incorporating ChatGPT into your data strategy represents a forward-thinking approach to business intelligence. It equips your business with advanced tools to make more informed, accurate, and strategic decisions. By embracing this AI-powered transformation, businesses can not only analyze their past and present with greater clarity but also anticipate and shape their future more effectively. The journey towards AI integration in data analysis is a step towards harnessing the full potential of your data, ultimately driving innovation and sustainable business growth.